Sports Analytics, Models and Algorithms – Quantitative betting strategies
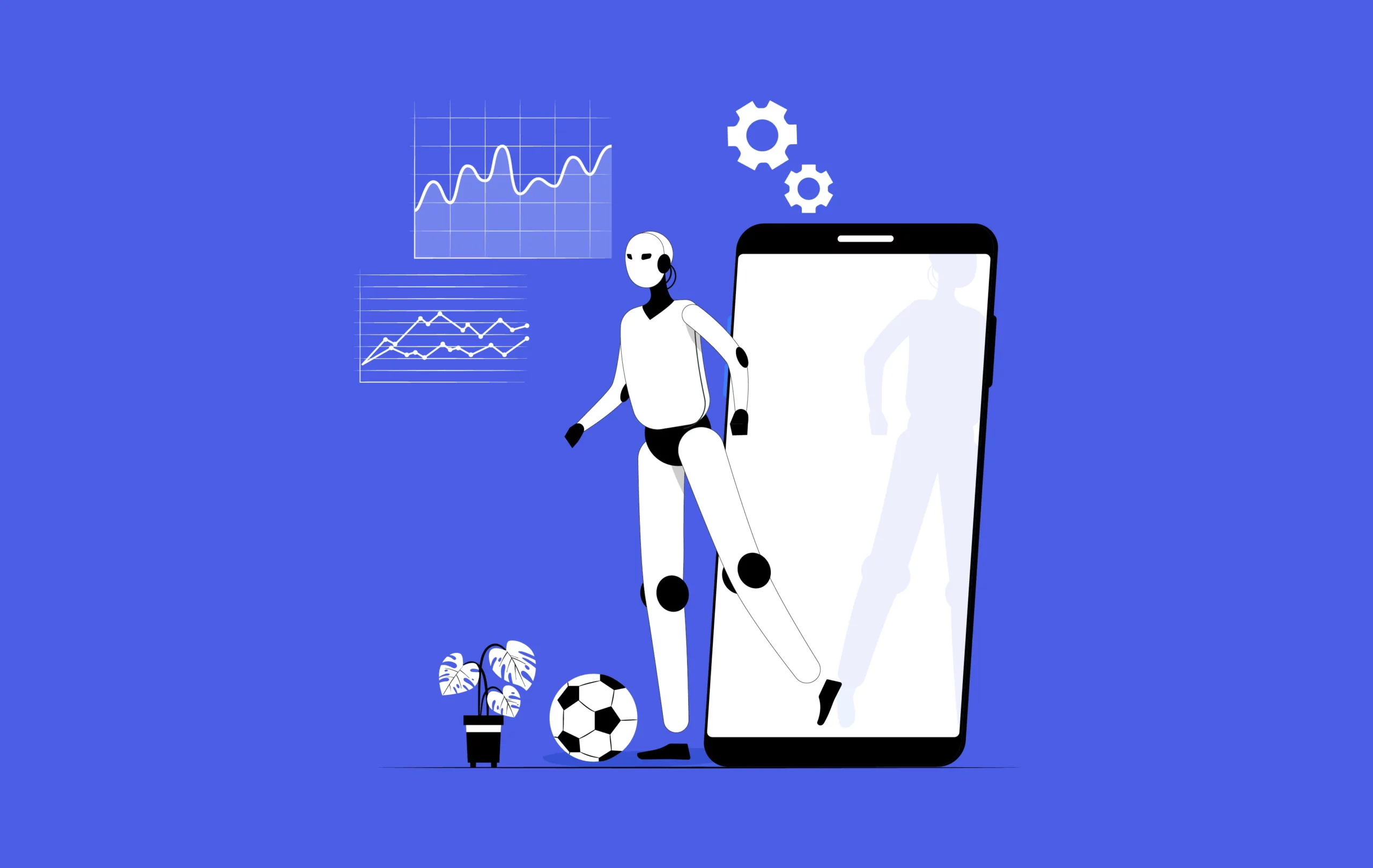
While subjective analysis and gut intuition influence many bettors, the use of data-driven models and algorithms has unlocked a new era of quantitative sports betting. Leveraging historical statistics, predictive analytics, simulations and machine learning can confer a valuable edge.
This guide will explain key sports betting modeling approaches, available tools, prudent usage tips and bankroll strategies to effectively incorporate predictive analytics into your quantitative betting process as a beginner.
Predictive Models
Sophisticated models use past game, team and player data to forecast future outcomes and probabilities. For example:
- Elo-based models dynamically update ratings and win odds.
- Regression models quantify relationships and interactions between variables.
- Machine learning algorithms uncover complex patterns.
Sharp books are already using such models, so gaining parity is crucial.
Where to Get Models
Many proven models exist across sports:
- Model providers like Sports Analytics, BetQL and Athlytix aggregate models into consensus picks.
- Some sportsbooks like Pinnacle offer proprietary models for subscribers.
- Modeling package like Skyll Sports enable building your own custom models.
- Follow expert quants sharing models, but validate quality.
Understand Model Assumptions
Be aware of the limitations and inherent biases in modeling based on data inputs and methodologies used. No model can capture the full complexity of sports.
For example game simulations cannot account for sudden injuries. Bet against mispriced over-optimizations. Models complement analysis rather than replace it.
Algorithmic Betting
Algorithms can automate and optimize parts of the betting process like data collection, line shopping, bet placement and bankroll management.
Algorithms lack discretion so safeguard against blind usage. But they excel at speed and finding patterns humanly impossible to discern in vast data.
Getting Started with Model Implementation
When using models:
- Start with basic models like logistic regression rather than advanced AI to understand key drivers.
- Bet smaller units as you assess predictive performance across a representative sample of games.
- Tweak models by adding significant variables and removing ineffective factors.
- Complement models with your own subjective analysis.
The more data fed into models, the better they optimize. But beware overfitting based solely on past results.